Global AI-Powered Smart Traffic Regulation
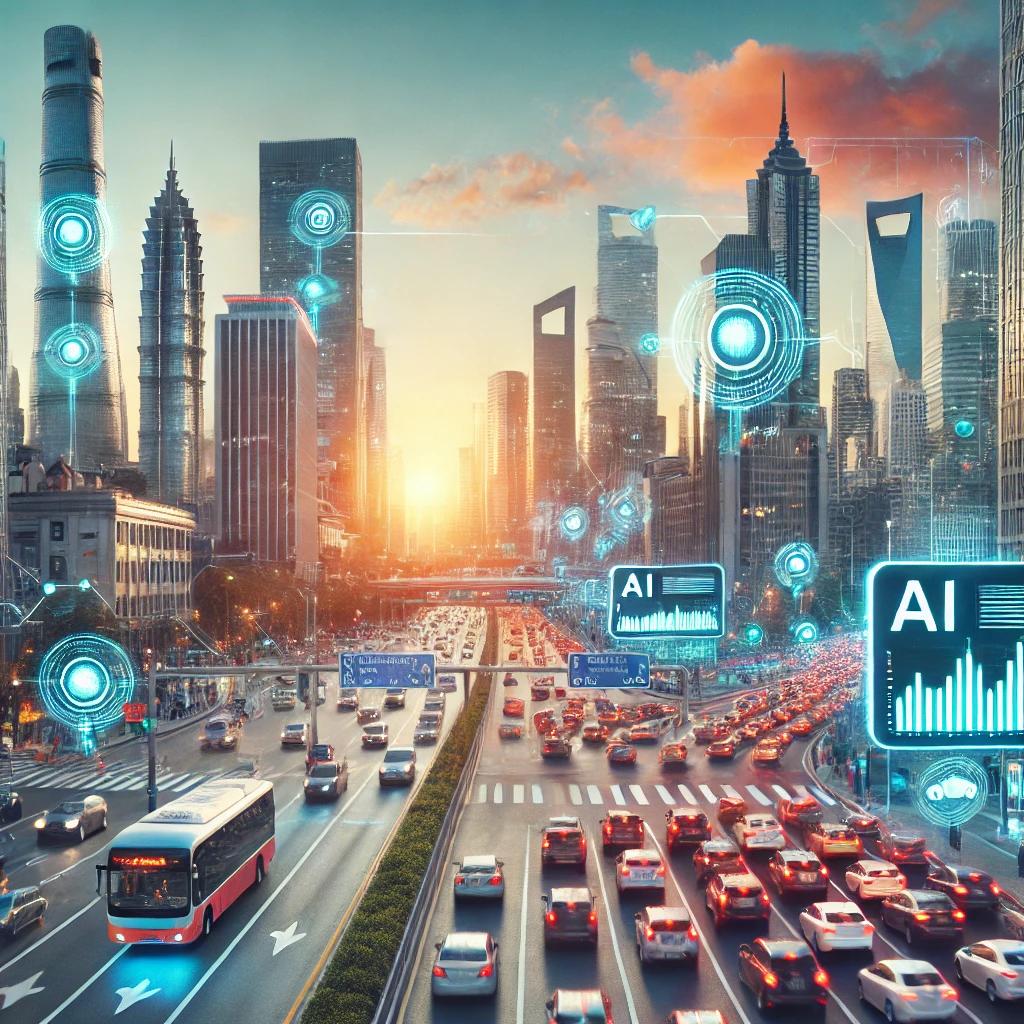
Introduction
Overview of the Startup Idea: Regulating traffic globally using predictive AI and large language models (LLMs) to enhance infrastructure utilization and predict future stress.
Purpose and Benefits: Improve traffic flow, reduce congestion, and optimize infrastructure investments by leveraging advanced AI technologies, benefiting cities worldwide.
Problem Statement
Description of the Problem: Urbanization and increasing vehicle ownership worldwide lead to severe traffic congestion, infrastructure stress, and inefficient use of public transportation. Major cities like New York, Tokyo, London, and Mumbai face significant traffic challenges, affecting daily commutes and economic productivity.
Current Solutions and Gaps: Existing traffic management systems in many cities lack predictive capabilities and often fail to address future growth and migration patterns. Traditional methods focus on reactive measures rather than proactive solutions.
Solution
Detailed Description of the Idea: Utilize AI and LLMs to analyze real-time traffic data, predict peak hours, and forecast future infrastructure stress. Incorporate migration patterns to optimize public transportation networks and anticipate changes in traffic flow.
Unique Selling Proposition (USP): Combining AI with LLMs for comprehensive traffic regulation, considering both daily fluctuations and long-term growth patterns. The solution adapts to the unique traffic conditions and migration patterns of different cities globally.
How It Addresses the Problem: By predicting traffic trends and future stress points, the solution enables proactive measures, reducing congestion and optimizing infrastructure use. This helps city planners and authorities make informed decisions to enhance urban mobility.
Market Analysis
Market Size and Growth Potential:
- The global intelligent transportation systems market is expected to reach USD 63.66 billion by 2025, growing at a CAGR of 10.5%.
- Increasing urbanization and smart city initiatives drive the demand for advanced traffic management solutions.
Target Audience and Demographics:
- Government agencies and urban planners in major cities worldwide.
- Public transportation authorities looking to optimize networks.
- Private sector companies involved in smart city projects.
Market Trends and Insights:
- Growing investment in smart city projects and urban infrastructure.
- Increasing adoption of AI, IoT, and big data analytics in traffic management.
- Enhanced focus on sustainable urban mobility solutions.
Competitive Landscape
Key Competitors:
- Waze (Google): Provides real-time traffic data.
- IBM Traffic Prediction Tool: Offers predictive analytics for traffic management.
- Siemens Intelligent Traffic Systems: Delivers comprehensive traffic management solutions.
Competitive Analysis (Strengths and Weaknesses):
- Waze provides real-time data but lacks comprehensive predictive capabilities.
- IBM offers robust predictive analytics but may not cater to the specific needs of every city.
- Siemens has a strong presence in traffic management but may require significant investment for implementation.
Differentiation Strategy:
- Tailored for diverse traffic conditions in different cities globally.
- Integration of LLMs for nuanced predictions based on local language data and cultural contexts.
- Scalable and adaptable solution for varying city sizes and complexities.
Business Model
Revenue Streams:
- Subscription-based model for government agencies and urban planners.
- Licensing fees for public transportation authorities.
- Consultancy services for infrastructure planning and optimization.
Pricing Strategy:
- Tiered pricing based on city size and complexity.
- Custom pricing for large-scale projects and long-term contracts.
Cost Structure:
- Development and maintenance of AI and LLM models.
- Data acquisition and storage.
- Marketing and sales expenses.
Marketing and Sales Strategy
Go-to-Market Plan:
- Collaborate with smart city initiatives and government bodies globally.
- Showcase pilot project successes at international industry conferences and forums.
Customer Acquisition Strategy:
- Direct sales to government agencies and urban planners.
- Partnerships with public transportation authorities and infrastructure development firms.
Sales Channels and Partnerships:
- Direct sales team for government and B2B clients.
- Strategic partnerships with global infrastructure development firms and technology providers.
Risks and Challenges
Potential Risks:
- Data privacy and security concerns in different regions.
- Resistance from traditional traffic management bodies and public skepticism.
Mitigation Strategies:
- Implement robust data security measures complying with local regulations.
- Conduct awareness campaigns highlighting the benefits of AI-driven traffic regulation and successful case studies.
Conclusion
Summary of the Opportunity: Leveraging AI and LLMs for traffic regulation presents a significant opportunity to improve urban mobility globally, enhancing infrastructure utilization and reducing congestion in major cities around the world.
Next Steps for Validation and Development:
- Conduct feasibility studies and gather stakeholder feedback.
- Develop MVP and initiate pilot projects in select cities worldwide.